In PCA the. Figure 1 will be used as an example to explain and.
Linear Discriminant Analysis Lda Vs Principal Component Analysis Pca Youtube
Here I avoid the complex linear algebra and use illustrations to show you what it does so you will k.
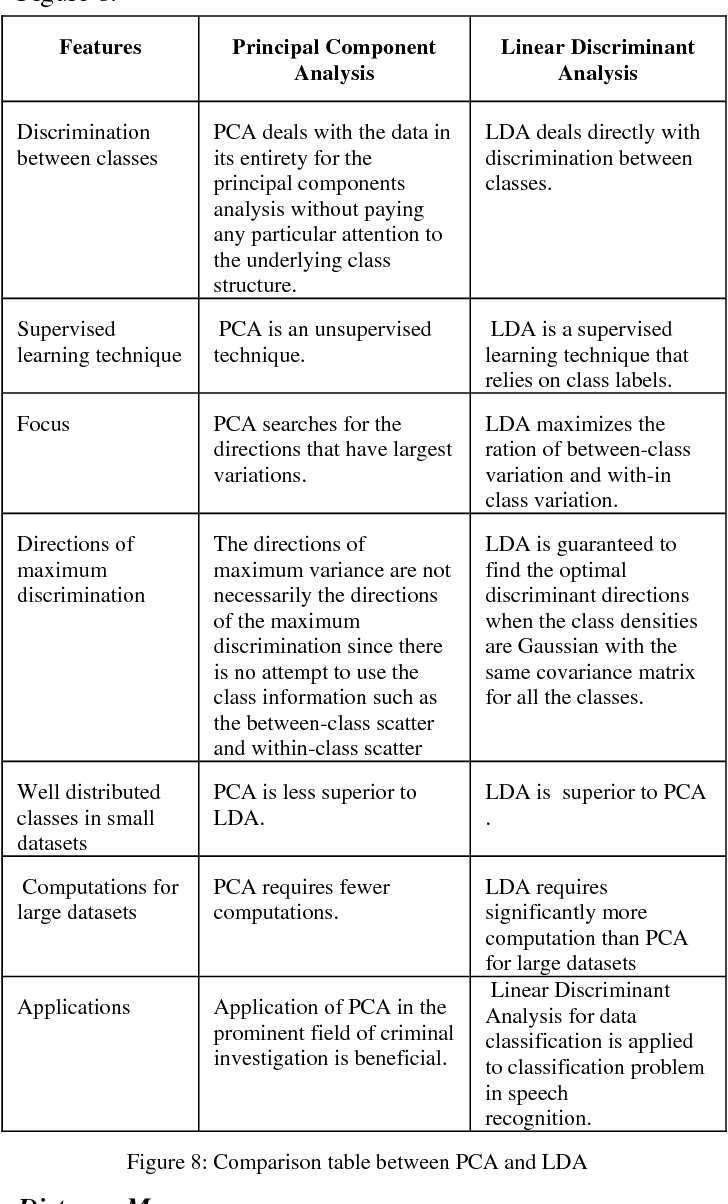
. In the PCA graph you can visualize the whole dataset properly differentiate well among the classes. A simple way of viewing the difference between PCA and LDA is that PCA treats the entire data set as a whole while LDA attempts to model the differences between classes. Whereas in the LDA graph the classes are not clearly separable due to the.
LDA is a supervised whereas PCA is unsupervised and ignores class labels. We can picture PCA as a technique. The prime difference between LDA and PCA is that PCA does more of feature classification and LDA does data classification.
Unlike PCA LDA is a supervised learning algorithm wherein the purpose is to classify a set of data in a lower dimensional space. LDA topic modeling discovers topics that are hidden latent in a set of text documents. By doing this a large chunk.
LDA on the other hand tries to explicitly model difference between classes labels within the data. Both LDA and PCA are linear transformation techniques. It does this by inferring possible topics based on the words in the documents.
The LDA models the difference between the classes of the data while PCA does not work to find any such difference in classes. In PCA the factor analysis builds the feature. In LDA we search for latent variables that.
The principal components PCs for predictor variables provided as input data are estimated and. We are going to. Both LDA and PCA are linear transformation techniques.
LDA is a classification method while PCA is a hierarchically optimized feature generation. Here we are using Iris dataset and K-NN classifier. LDA tries to maximize the separation of known categories.
Hi in this post I am gonna explain how dimensionality reduction techniques affect the prediction model. In addition to the above LDA is a supervised method. Data from FTIR or other spectral instruments analysed by different chemometric methods.
There are three several steps of this analyses first step is pre-processing of spectral. PCA looks for attributes with the most variance. Principal Component Analysis or PCA is a dimensionality-reduction method that is often used to reduce the dimensionality of large data sets by transforming a large set of.
LDA is surprisingly simple and anyone can understand it. Linear Discriminant Analysis LDA using Principal Component Analysis PCA Description. Which means that - in contrast to the PCA for example - you predefine groups of objects.
LDA is like PCA both try to reduce the dimensions. In your question it seems that LDA and PCA are methods to solve the same problem and they are not. Both techniques are projecting the data onto a smaller feature subspace.
The main difference is that PCA is label agnostic -- it treats the entire data set as a whole. With PCA I would find the directions components that maximize the variance in the dataset without. What is the difference between LDA and PCA for dimensionality reduction.
You dont see much of a difference here because the first 2 components of the PCA captures most of the variance in the iris dataset. I actually plot the different rows of the. LDA is a supervised whereas PCA is unsupervised PCA.
In other words the objective is to create a. Unlike PCA LDA is a supervised learning algorithm wherein the purpose is to classify a set of data in a lower dimensional space. Principal Components Analysis PCA is an algorithm to transform the columns of a dataset into a new set of features called Principal Components.
Illustrative Example Of Principal Component Analysis Pca Vs Linear Discriminant Analysis Lda Is Pca Good Guy Or Bad Guy By Gopi Sumanth Analytics Vidhya Medium
Dimensionality Reduction Pca And Lda With Practical Implementation By Amir Ali Wavy Ai Research Foundation Medium
Face Recognition Using Pca Lda And Various Distance Classifiers Semantic Scholar
Pca Vs Lda In Different Learning Scenarios Left Given A Large Size Download Scientific Diagram
0 Comments